How Physics can greatly make dynamic AI solutions
Some of us may remember an old TV ad, where a kid is guiding a car driver back into a parking lot.
image for illustrative purpose
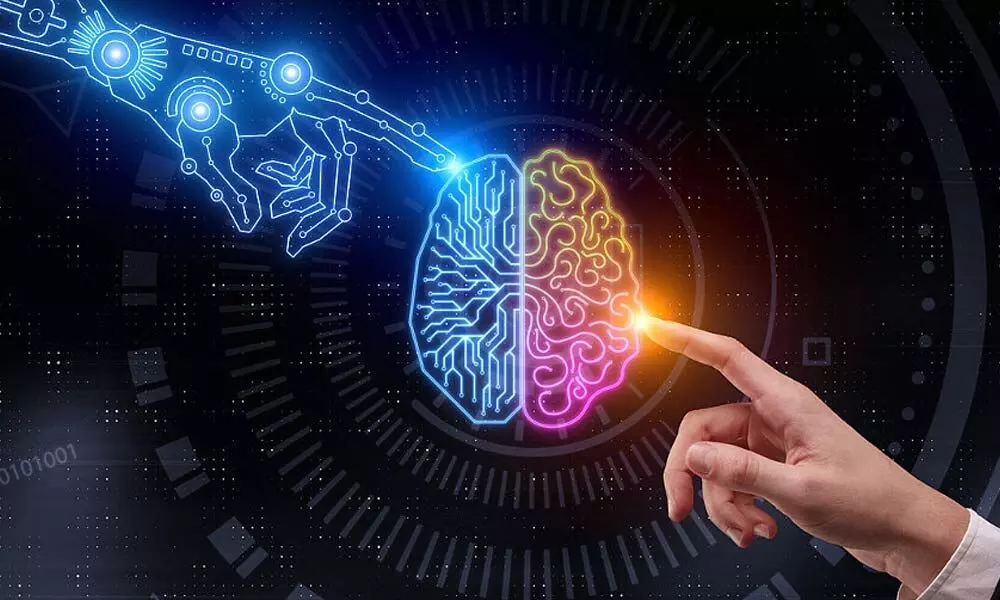
Some of us may remember an old TV ad, where a kid is guiding a car driver back into a parking lot. As the driver gradually inches into the parking lot, he keeps checking with the boy if the rear hit the wall yet or not, and the boy keeps responding, 'not yet'. All of a sudden, the back of the car rams into the wall. The boy yells in celebration, "Yes, now it hit the wall." The humor in the situation brings out the point that feedback without understanding the essence of an exercise is pointless.
There are plenty of AI systems built today that go purely by the predictive mathematics behind it and the training data fed to them. They do not take the mechanics of the physical world into account in predicting outcomes. The users get baffled by programmers' inability to explain what's happening in their AI's digital brain. While this system works well in most situations, they do not know how it arrived at that solution. How can we trust these models in critical situations? That's a very legitimate question, especially if these models are to be integrated into complex systems like mass transportation or a nuclear reactor operation, where complicated, unforeseen situations might occur at any time - and could result in loss of lives.
Data scientists are now going beyond depending on traditional neural networks - which means comparing to ML data alone. They are integrating principles of Physics into their models. Physics constrains the problem, and if the neural network produces an unrealistic result, it gets flagged so that the system avoids such mistakes in the future. This new trend is dramatically boosting AI's ability to produce accurate results.
For instance, one famous institute that predicts hurricane movements using AI relies on a neural network alongside well-understood physics: They know how the hurricanes slow down or accelerate, how water evaporation affects them, how ocean currents move, how much the Sun is warming the Earth, how the cyclone moves across the ocean, and whether it would hit the land or fizzle out. Importantly, they can explain the outcomes based on science and rational thinking.
Physics and machine learning have some similarities. Both disciplines focus on making accurate observations, and both build models to predict future outcomes. One of the terms that physicists often use is co-variance which means that physics should be independent of which rule is used or what kind of observers are involved. No different than what an AI data modeler would look for.
PINNs, for 'Physics-Informed Neural Networks', are a new class of neural networks combining machine learning and physics. The inventors of PINNs define them as 'neural networks that are trained to solve supervised learning tasks while respecting any given law of physics described by certain complex mathematical equations'.
Even within the PINN networks, the behaviour depends on different fields (such as mechanics, electromagnetism or thermodynamics) based on its application. Each has its laws and models, which constitute the key ingredient of the framework being built. The idea of PINNs is to 'encode' these laws of physics and scientific knowledge into the learning algorithms to make them more robust.
One such real-world application is to create the digital twin of an aero-plane, and simulation software using PINNs creates the most realistic flight environment and their mutual interaction. This approach can be applied to various areas such as climate prediction, astronomy, material science, or predicting earthquakes. Some MIT researchers claim that they used machine-learning techniques to detect extrasolar planets and gravitational waves from colliding black holes.
With physics in AI, I have no doubt that the evolution map of the applications of AI is definitely headed in the right direction. Physics has evolved over centuries where great scientists and philosophers observed nature, derived unique insights and put them down in a mathematical form for later generations to use. Maybe the time has come for AI data scientists to embed the same into their framework.